AI-Powered Sound Analysis for Anomaly Detection in Manufacturing
With AI, manufacturers can preempt equipment failures and ensure regulatory compliance...
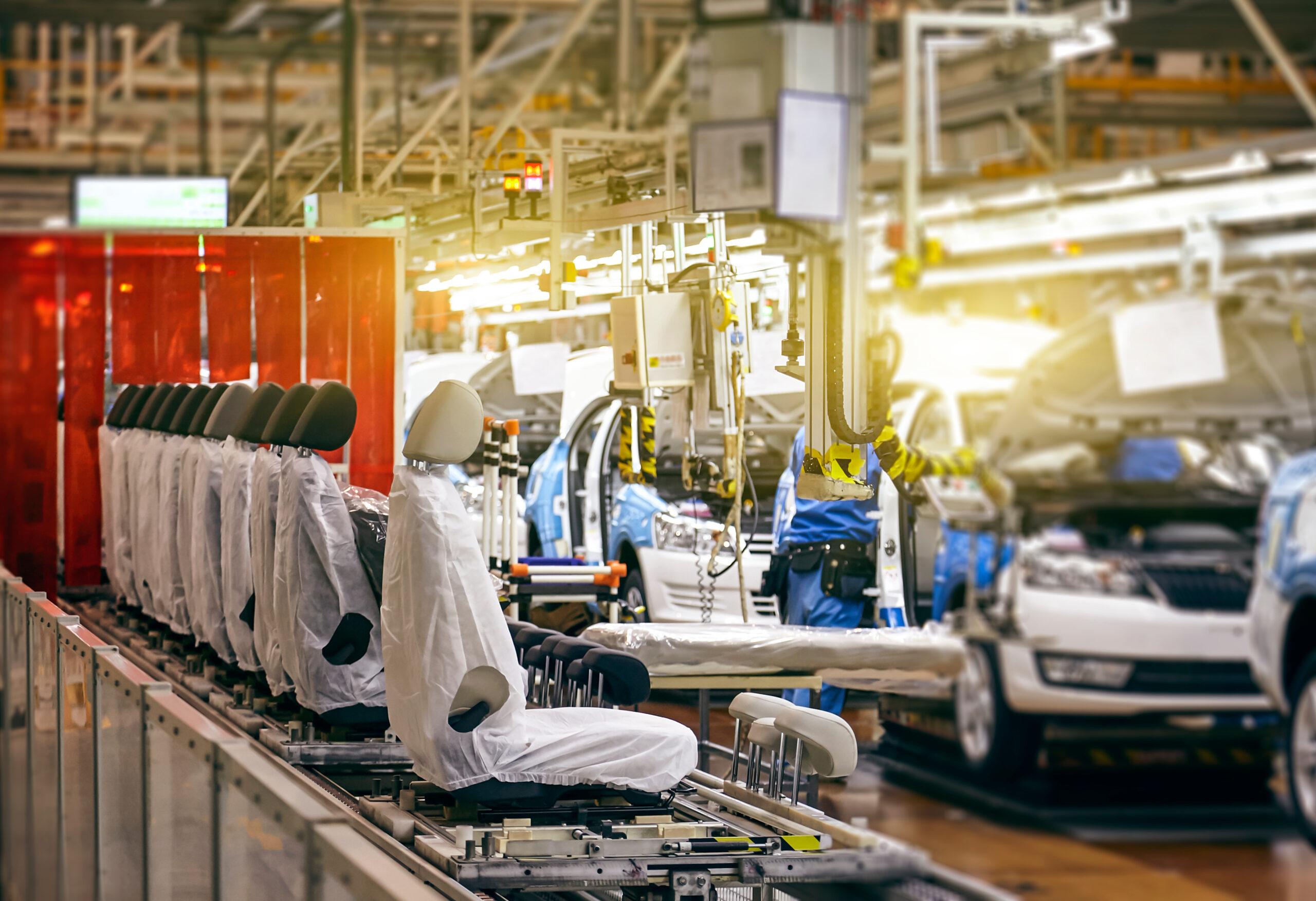
Businesses often grapple with the financial and operational fallout from regulatory issues or equipment failures, typically stemming from systemic rather than individual failures. Pinpointing these underlying problems can be challenging due to missed signals, but AI-driven Anomaly Detection offers a powerful solution.
Condition monitoring aims to prevent equipment failures by analysing machine parameters like vibration and sound to identify errors early.
Traditional monitoring methods, however, struggle to sift through today’s massive data volumes, making anomaly detection difficult.
Incorporating machine learning into monitoring processes is crucial for improving productivity and avoiding issues. Advanced techniques are essential in our data-rich industrial environments. McKinsey research shows that data-driven strategies can reduce machine downtime by 30-50% and increase machine life by 20-40%.
What Is Anomaly Detection?
Anomaly Detection zeroes in on data events deviating from the norm. Early detection of these anomalies can preempt issues, bolstering safety, security, and efficiency across various sectors.
Sound patterns can reveal problems before they lead to failure or require costly repairs. In manufacturing, anomaly detection is vital for adherence to regulatory standards. Anomalies could signal significant production bottlenecks, leading to diminished throughput, increased downtime, and compromised product quality.
The Challenge: Anomaly Detection in Sound
Identifying anomalies in sound data presents unique challenges due to its inherent complexity and high dimensionality. Sound data encompasses a continuous flow of audio signals varying in frequency, amplitude, and duration, compounded by background noises that obscure anomalies.
Manual detection efforts are further hampered by the subjective nature of human perception regarding sound, making consistent anomaly identification challenging even for experts.
The Solution: Anomaly Detection APIs for Audio
Training AI models on extensive sound datasets enables these algorithms to identify sound patterns and anomalies that might elude human detection. These models offer high accuracy and consistency, crucial for early anomaly detection and proactive measures.
Benefits of automated anomaly detection include:
Quality Control: AI can identify production anomalies by monitoring for unusual sounds, signalling defective components during manufacturing.
Predictive Maintenance: Early detection of sound pattern changes can indicate impending equipment failure, allowing for timely maintenance and reducing operational interruptions.
Environmental Monitoring: Unusual sounds or noise levels can indicate leaks, emissions, or hazardous conditions, aiding in accident prevention and safety enhancement.
Security Monitoring: In public or commercial settings, detecting abnormal sounds can signal security breaches, facilitating rapid response.
Cost Savings: Early issue identification through anomaly detection minimises the costs associated with defects, waste, and equipment downtime, while optimizing production efficiency.
Introducing Risso’s Anomaly Detection for Industrial Sounds
Risso has curated an extensive model zoo featuring pre-trained machine learning models tailored for industrial sound anomaly detection. Our manufacturing-focused models are particularly invaluable for identifying potential production process issues before they escalate into significant problems.
Our datasets comprise thousands of audio recordings capturing specific industrial equipment or processes in various states. For instance, our Pump dataset includes sounds from pumps under normal conditions, as well as clogged or damaged states. Similarly, our Valve dataset encompasses sounds from valves in different operational and wear conditions.
Utilising these pre-trained models enables manufacturers to seamlessly integrate anomaly detection into their production workflows, enhancing efficiency and product quality.
Integrating AI technologies like Risso’s into manufacturing aligns with the broader movement towards Industry 4.0, revolutionising industrial processes through automation and data exchange. By adopting Risso’s Anomaly Detection AI, manufacturers can not only enhance product quality and operational efficiency but also gain critical insights for ongoing improvement and innovation, maintaining a competitive edge in the rapidly evolving market.
Subscribe to our newsletter
See why business of all sizes use Risso AI for sound analysis